Introduction
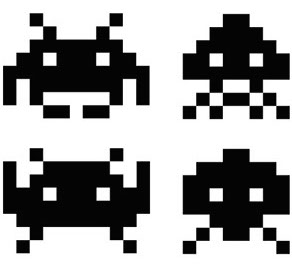
We are a theoretical group
interested
in the collective behavior of
physical and biological systems in which disorder and strong
interactions play an important role.
We specialize in statistical mechanics, soft condensed matter,
evolutionary biology and ecology. We are particularly interested in questions surrounding the arrow of time, such as how materials and organisms fall apart, how evolution tangles ecological relationships, how our memory of the past fades, and how predicting the future relates to retrodicting the past. Below is a brief description of some of our recent work.
Aging, Failure and
Death
Standard theories of
aging typically focus on microscopic mechanisms such as oxidative
damage or telomere shortening. However we age and die not because we
run out of cells, but because small scale failures on the cellular
level manifest at larger scales, leading to a macroscopic catastrophe.
We
view aging as an emergent universal property of systems that consist
of a large number of interdependent components (read).
The video below shows how a large number of
interdependent nodes fail.
Failure
can also be viewed as a microscope that reveals the structure of a
complex system. The failure times of components informs how they are
interconnected. Read.
Recently,
our experimental collaborators verified the interaction based picture
of aging in synthetic tissues. Read.
Based
on experimental observations and earlier theoretical ideas, we predict
that tissues collapse through a wave of failure that propagates from
outside inwards, with non-monotonic velocity. Read.
We
organized a nice workshop surrounding
these topics.
Disordered
Materials
Materials
such as glasses, polymers, disordered crystals, quasi-crystals and
proteins lack long range order, and are thus referred as disordered
solids. The standard model describing disordered solids at low
temperatures postulate that these materials are composed of two level
systems, i.e. atoms, or
groups of atoms with two available discrete configurations.
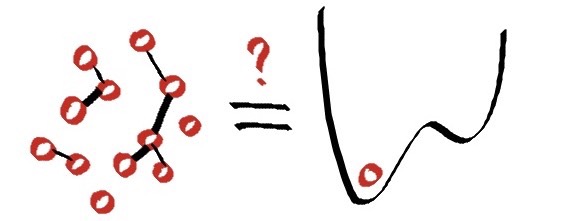
All disordered solids universally
exhibit
certain properties which cannot be explained by the two level systems
model. Here
is a critique of the
said model, and here is a more general model, from which the universality
is derived.
|
Evolution of Cooperation
We
develop physical models of evolutionary game theory to understand how
cooperation emerges and persists despite the evolutionary incentive to
cheat. Recently we discovered
that
flow shear enables and promotes
social behavior in microbes. Specifically, shear tears apart social
communities clusters,
and thereby limiting the spread of cheating strains. The videos above
shows how flow patterns can shape social evolution. In a vortex,
cooperative microbes can sustain only within an annular region. In a
flowing pipe,
they can only sustain near the boundaries, where the shear is larger
than a critical amount. Everywhere else cheaters take over, causing the
groups to weaken and die. Read.
We also found that the physical characteristics such as diffusion
lengths, flow patterns, and molecular decay rates play a significant
role in governing whether a microbial community will evolve specialist
or generalist forms of cooperation. Read.
Although
cheating behavior is often devastating for social species, we have
discovered that in special cases, cheating can, counterintuitively,
benefit the community. This will happen, in the context of a disease or
tumor, where rapid growth can be beneficial. Cheaters fueled by
altruists will expand rapidly, and overwhelm the host immune system. Read.
Rewinding Randomness
Many
astonishing facts about the origin of the universe, evolution of life,
or history of civilizations will never be directly observed, but will
only be inferred in the light of their manifestations in the present.
Forward in time, any state of knowledge, regardless of how exact, will
invariably deteriorate into an entropy maximizing probability
distribution. We adresss the converse question: Given a measurement at
present time, how rapidly does our knowledge fade away backwards in
time? Read.
Theoretically
relevant information such as original causes or underlying mechanisms
are seldom directly observable. For example, one can easily measure the
amount of proteins expressed in a cell but not necessarily know what
interactions lead to the particular expression pattern. One can observe
a certain species decline in numbers, but not exactly know which
ecological relationship, or what triggering event causes the decline.
When a virus spreads seemingly spontaneously, or when an invasive
species expands its range, it is very difficult to know where it
exactly came from, or its evolutionary trajectory that lead to its
final success.
We develop algorithms and formulae to infer causal
origins from raw empirical data, such
as identifying the original trigerring event given an observed final
state. Read.
Entropic Forces
When
two macromolecules come very near each other in a fluid, the
surrounding fluid molecules, having finite volume, are less likely to
get in between. This causes the macromolecules to apprach each other as
if attracted to one other. This entropic force is called depletion.
Here we calculate the depletion force between linear disordered
macromolecules. Read.
Slow Demons
A
Maxwell's demon is a hypothetical device that opens and closes a gate
separating two chambers filled with gas, based on its observations of
approaching gas molecules. This way it sorts the atoms and reduces the
entropy of the system. This thought experiment has been instrumental
in establishing the connections between information, computation and
thermodynamics for over a century.
Here
we take into consideration the finite response time and finite size of
the demon. For example, the gate cannot operate faster than the speed
of light. These limitations establish theoretical upper limits toheat
and mass transfer via local information processing. Read.
|
Ecological
Evolution
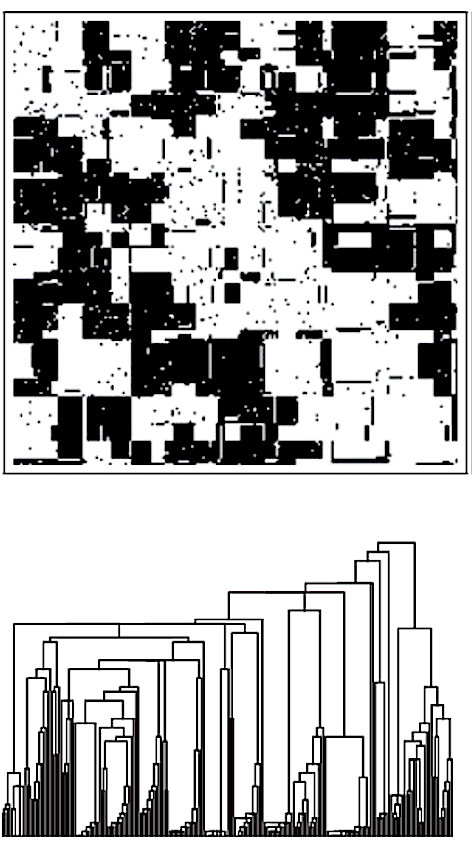 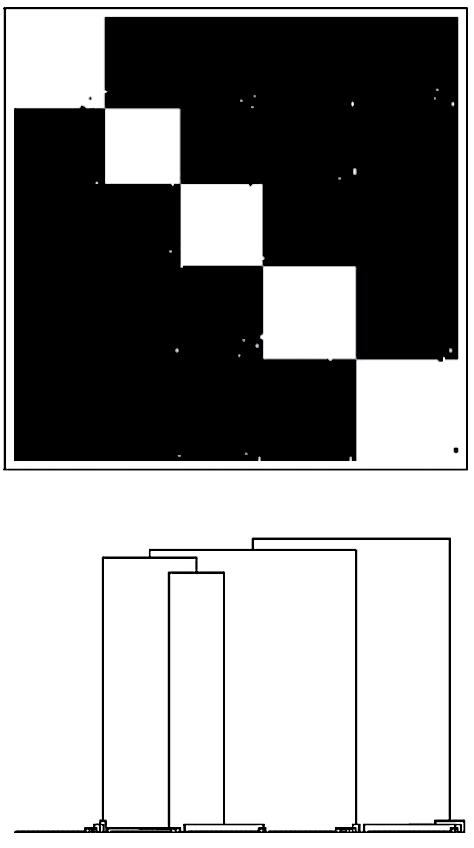
Start
with a
large number
of unrelated species, put them together
and wait for many generations. Eventually the interactions between them
evolve into a tangled web of exchanges - so much, that no species can
survive in isolation. Above are connectivity matrices and phylogenetic
trees of two such communities subject to different selective pressures.
Higher pressures (right) leads to the formation of specialized
communities. Read.
Model
Awareness
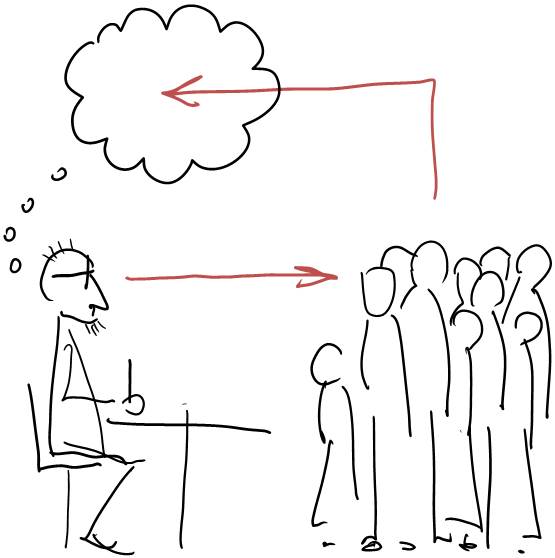
A
model need not be a passive descriptor of its
subject. If the subject is affected by the model building process, the
model must be updated in real time. In some cases, the very act model
building can cause the otherwise correct model to fail, or cause an
otherwise incorrect model to succeed. In other
cases, attempting to predict the future can be detrimental to the
predictor. Read.
We
studied the dynamics of a predictive swarm aiming to maximize the
uptake of some resource. A predictive agent tries to estimate what
others will do, and update its behavior accordingly. All other
predictive agents are doing the same, trying to predict the trajectory
of agents that are trying to predict them! The video
above shows a few iterations o the thoughts of a predictor, while it says “they know
that I know that they know that I know...". On the
left, the resources are initialized
randomly, whereas on the right resources are concentrated in two spots,
one large and one small. Read.
Active
Inference
Scientific inference involves
obtaining the unknown properties or behavior of a system in the light
of what is known, typically, without changing the system. We propose an
alternative to this approach: a system can be modified in a targetted
way, preferrably by a small amount, so that it becomes more predictable
or more retrodictable. Read.
Quantization of
Temperature
Temperature
is conventionally defined in terms of the number of “possible" microstates,
given a set of macroscopic constraints such as total energy or
particle number. It is a remarkable that an information theoretical
quantity can
connect so well to physical observables, such as the hight of a mercury
collumn, or the volume of a baloon.lead to its
final success.
Here we offer an alternative view of temperature, and
propose that
temperature must be reformulated as a non-local non-realistic quantum
variable. We demonstrate that if a large but finite system is split
into two subsystems, their temperatures must get entangled. As such, an
operator description of temperature becomes necessary to avoid an
EPR-type causality violation. In this new picture, temperature is
subject to the constraints of quantum mechanics, where its measurement
must necessarily accompany a wavefunction collapse into a "temperature
eigenstate". Read.
|